
The report of Intergovernmental Panel on Climate Change (IPCC) shows that 20–30% of total greenhouse gases (GHGs) are released from urban transportation operation including passenger and freight transportation. The results not only show the effectiveness of the proposed approaches in estimating fuel consumption/emissions but also indicate their advantages for uncovering the relationships between fuel consumption and vehicles’ activities in road networks.Īs urbanization accelerates, transport-related environmental issues deteriorate. We also analyze the types of activities that produced fuel consumption on each road segment to explore the patterns and mechanisms of fuel consumption in the study area.

The estimation accuracy of the proposed approach is 88.6%. In the case study, fuel consumption and emissions of a single vehicle and a road network are estimated and visualized with GPS data. For each STPS, fuel consumption, hot emissions, and cold start emissions are estimated based on activity type, i.e., MA, SA with engine-on and SA with engine-off. Then we present an N-Dimensional framework for estimating and visualizing fuel consumption/emissions. First, we build space-time paths of individual vehicles, extract moving parameters, and identify MA and SA from each space-time path segment (STPS). By adopting the analytical construct of the space-time path in time geography, this study proposes methods that more accurately estimate and visualize vehicle energy consumption/emissions based on analysis of vehicles’ mobile activities ( MA) and stationary activities ( SA). Previous studies, which estimated fuel consumption/emissions from traffic based on GPS sampled data, have not sufficiently considered vehicle activities and may have led to erroneous estimations.
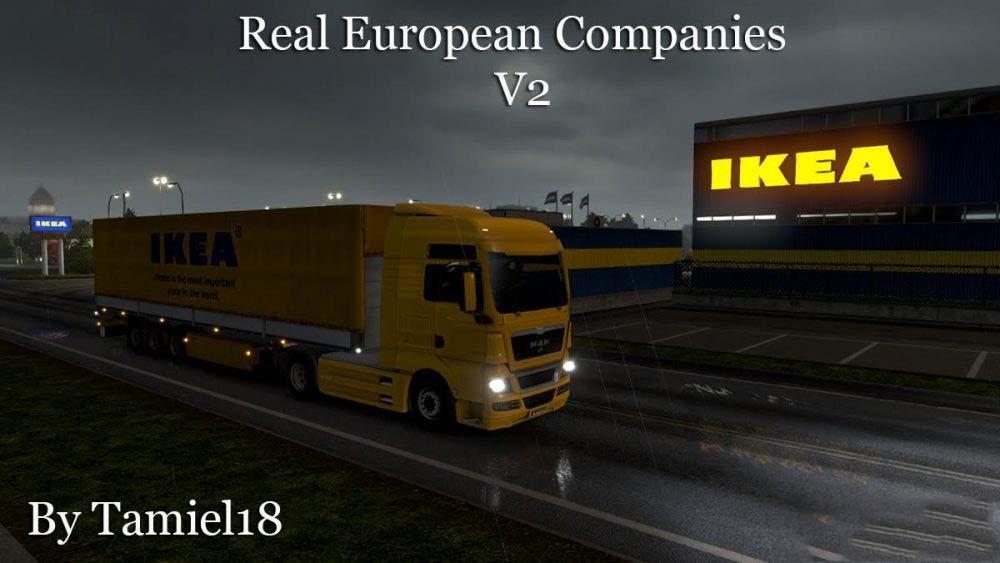
Analysis of GPS big data collected from vehicles can provide useful insights about the quantity and distribution of such energy consumption and emissions. The energy consumption and emissions from vehicles adversely affect human health and urban sustainability.
